Highlights
- Software development research is divided into two incommensurable paradigms.
- The Rational Paradigm emphasizes problem solving, planning and methods.
- The Empirical Paradigm emphasizes problem framing, improvisation and practices.
- The Empirical Paradigm is based on data and science; the Rational Paradigm is based on assumptions and opinions.
- The Rational Paradigm undermines the credibility of the software engineering research community.
Very good paper by @[email protected] discussing Rational Paradigm (non emprirical) and Empiriral Paradigm (evidence-based, scientific) in software engineering. Historically the Rational Paradigm has dominated both the software engineering research and industry, which is also evident in software engineering international standards, bodies of knowledge (e.g. IEEE CS SWEBOK), curriculum guidelines, ... Basically, much of the "standard" knowledge and mainstream literature has no basis in science, but "guru" knowledge. But people rarely follow rational approaches successfully or faithfully, which suggest using detailed plans, ...
It also argues that currently software engineering is at level 2 in a "informal scale of empirical commitment". In comparison, medicine is at level 4 (greatest level in empirical commitment).
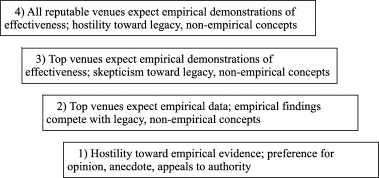
I think SE is at level two. Most top venues expect empirical data; however, that data often does not directly address effectiveness. Empirical findings and rigorous studies compete with non-empirical concepts and anecdotal evidence. For example, some reviews of a recent paper on software development waste [168] criticized it for its limited contribution over previous work [169], even though the previous work was based entirely on anecdotal evidence and the new paper was based on a rigorous empirical study. Meanwhile, many specialist and second-tier venues do not require empirical data at all.
And concludes with some implications
-
Much research involves developing new and improved development methods, tools, models, standards and techniques. Researchers who are unwittingly immersed in the Rational Paradigm may create artifacts based on unstated Rational-Paradigm assumptions, limiting their applicability and usefulness. For instance, the project management framework PRINCE2 prescribes that the project board (who set project goals) should not be the same people as project team (who design the system [108]). This is based on the Rationalist assumption that problems are given, and inhibits design coevolution.
-
Having two paradigms in the same academic community causes miscommunication [4], which undermines consensus and hinders scientific progress [171]. The fundamental rationalist critique of the Empirical Paradigm is that it is patently obvious that employing a more systematic, methodical, logical process should improve outcomes [7], [23], [119], [172], [173]. The fundamental empiricist critique of the Rational Paradigm is that there is no convincing evidence that following more systematic, methodical, logical processes is helpful or even possible [3], [5], [9], [12]. As the Rational Paradigm is grounded in Rationalist epistemology, its adherents are skeptical of empirical evidence [23]; similarly, as the Empirical Paradigm is grounded in empiricist epistemology, its adherents are skeptical of appeals to intuition and common sense [5]. In other words, scholars in different paradigms talk past each other and struggle to communicate or find common ground.
-
Many reasonable professionals, who would never buy a homeopathic remedy (because a few testimonials obviously do not constitute sound evidence of effectiveness) will adopt a software method or practice based on nothing other than a few testimonials [174], [175]. Both practitioners and researchers should demand direct empirical evaluation of the effectiveness of all proposed methods, tools, models, standards and techniques (cf. [111], [176]). When someone argues that basic standards of evidence should not apply to their research, call this what it is: the special pleading fallacy [177]. Meanwhile, peer reviewers should avoid criticizing or rejecting empirical work for contradicting non-empirical legacy concepts.
-
The Rational Paradigm leads professionals “to demand up-front statements of design requirements” and “to make contracts with one another on [this] basis”, increasing risk [5]. The Empirical Paradigm reveals why: as the goals and desiderata coevolve with the emerging software product, many projects drift away from their contracts. This drift creates a paradox for the developers: deliver exactly what the contract says for limited stakeholder benefits (and possible harms), or maximize stakeholder benefits and risk breach-of-contract litigation. Firms should therefore consider alternative arrangements including in-house development or ongoing contracts.
-
The Rational Paradigm contributes to the well-known tension between managers attempting to drive projects through cost estimates and software professionals who cannot accurately estimate costs [88]. Developers underestimate effort by 30–40% on average [178] as they rarely have sufficient information to gauge project difficulty [18]. The Empirical Paradigm reveals that design is an unpredictable, creative process, for which accounting-based control is ineffective.
-
Rational Paradigm assumptions permeate IS2010 [70] and SE2014 [179], the undergraduate model curricula for information systems and software engineering, respectively. Both curricula discuss requirements and lifecycles in depth; neither mention Reflection-in-Action, coevolution, amethodical development or any theories of SE or design (cf. [180]). Nonempirical legacy concepts including the Waterfall Model and Project Triangle should be dropped from curricula to make room for evidenced-based concepts, models and theories, just like in all of the other social and applied sciences.
Abstract
The most profound conflict in software engineering is not between positivist and interpretivist research approaches or Agile and Heavyweight software development methods, but between the Rational and Empirical Design Paradigms. The Rational and Empirical Paradigms are disparate constellations of beliefs about how software is and should be created. The Rational Paradigm remains dominant in software engineering research, standards and curricula despite being contradicted by decades of empirical research. The Rational Paradigm views analysis, design and programming as separate activities despite empirical research showing that they are simultaneous and inextricably interconnected. The Rational Paradigm views developers as executing plans despite empirical research showing that plans are a weak resource for informing situated action. The Rational Paradigm views success in terms of the Project Triangle (scope, time, cost and quality) despite empirical researching showing that the Project Triangle omits critical dimensions of success. The Rational Paradigm assumes that analysts elicit requirements despite empirical research showing that analysts and stakeholders co-construct preferences. The Rational Paradigm views professionals as using software development methods despite empirical research showing that methods are rarely used, very rarely used as intended, and typically weak resources for informing situated action. This article therefore elucidates the Empirical Design Paradigm, an alternative view of software development more consistent with empirical evidence. Embracing the Empirical Paradigm is crucial for retaining scientific legitimacy, solving numerous practical problems and improving software engineering education.